What is a Predictive Maintenance Schedule?
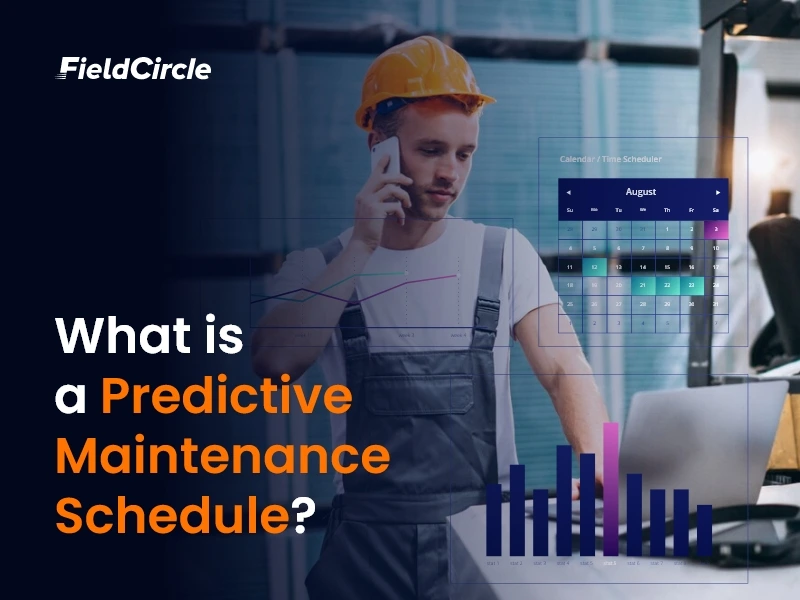
A Predictive Maintenance Schedule is a plan to forecast potential equipment failures through real-time data and predictive analytics and set up maintenance tasks before the problem occurs.
Predictive maintenance schedule follows a proactive approach where sensors continuously monitor key parameters like vibration, temperature, humidity, and pressure to identify early signs or anomalies. After that, it analyzes the data to predict and schedule the necessary maintenance to reduce downtime, minimize repair costs, and extend equipment lifespan.
Let’s explore the various facets of predictive maintenance and understand how it transforms maintenance from a chore to a strategic tool for operational excellence.
Why Implement a Predictive Maintenance Schedule?
Implementing a predictive maintenance schedule helps businesses optimize equipment performance, reduce costs, and improve operational efficiency. For that, it utilizes real-time data, advanced analytics, and condition monitoring technologies.
Here are the top 7 benefits of using a predictive maintenance schedule
- Reduce downtime through early issues detection by continuously monitoring equipment conditions such as vibration, temperature, and pressure.
- Extend asset lifespan by identifying early signs of equipment degradation and preventing wear-related failures.
- Optimize maintenance timing by reducing unnecessary work and minimizing resource waste.
- Minimize disruptions to production or operations by scheduling maintenance during off-peak hours.
- Lower maintenance costs by preventing equipment failures, costly emergency repairs, and unplanned downtime.
- Data-driven decision-making based on real-time data and advanced analytics to provide actionable insights into equipment health.
- Enhanced asset reliability and performance for better performance, higher production quality, and greater customer satisfaction.
What are the key components of a predictive maintenance schedule?
A predictive maintenance schedule depends on key components ranging from collecting and monitoring data to integrating maintenance activities with CMMS, ensuring a proactive and data-driven strategy. These critical components collect real-time data, analyze equipment usage and performance, and schedule timely interventions to prevent equipment and infrastructure failures.
- Data Collection and Monitoring
- Thresholds and Predictive Alerts
- Condition-Based Maintenance Triggers
- CMMS Integration
Real-time data collection is the core of a predictive maintenance schedule. Sensors on equipment and infrastructure help gather and monitor key performance indicators (KPIs) such as temperature, vibration, pressure, and humidity. These sensors are connected via the Internet of Things (IoT), enabling maintenance teams to seamlessly transfer data to predictive maintenance software, monitor equipment health, detect potential issues early, and intervene on time.
After collecting data, it is essential to set performance thresholds. The thresholds can be based on manufacturer specifications, such as the maximum operating temperature, historical performance data, such as the average vibration level before past failures, and industry best practices. When a parameter exceeds thresholds, the system sends a predictive alert as an early warning sign, enabling maintenance teams to take corrective actions before a minor issue turns into a significant failure.
Another critical component of the predictive maintenance schedule is condition-based maintenance triggers, which use real-time data to determine the optimal timing of maintenance activities. This method initiates maintenance only when machinery shows signs of potential failure. For example, oil quality sensors detect contamination and trigger a lubrication change. Likewise, if a machine’s vibration level exceeds the safe threshold, it prompts an inspection or a component replacement. This approach ensures that maintenance tasks are accurately performed on time, reducing unnecessary work while extending asset lifespan.
A Computerized Maintenance Management System (CMMS) is crucial for managing predictive maintenance workflows. Integrating CMMS allows businesses to automate maintenance schedules, track maintenance activities, generate work orders, and analyze KPIs such as equipment uptime, failure rates, and maintenance costs. By incorporating predictive maintenance data with a CMMS, companies build a centralized platform that streamlines data flow, enhances decision-making, and improves maintenance efficiency.
What Steps Are Involved to Develop a Predictive Maintenance Schedule?
The 11 steps involved in developing a predictive maintenance schedule that helps to develop a systematic approach for integrating data-driven insights, advanced monitoring technologies, and proactive maintenance strategies are as follows
- Identify Equipment for Predictive Maintenance
- Define Predictive Monitoring Parameters
- Install and Configure Condition Monitoring Tools
- Gather and Analyze Equipment Performance Data
- Set Condition Thresholds and Failure Limits
- Develop Predictive Models Using Analytical Tools
- Define Maintenance Tasks Triggered by Predictions
- Create and Optimize the Maintenance Schedule
- Integrate Predictive Maintenance with Existing Systems
- Train Maintenance Staff on Predictive Procedures
- Monitor KPIs and Adjust the Schedule Periodically
Firstly, it is essential to identify critical equipment that requires predictive maintenance and would benefit the most from this strategy. You should focus on assets with frequent breakdowns or costly repairs and high maintenance expenses. It also includes machines that support sensor integration and high-impact equipment. Some examples are production line machinery, HVAC systems in large buildings, or heavy-duty industrial equipment. Prioritizing high-risk assets helps businesses maximize ROI on their predictive maintenance program.
After identifying critical assets, it is time to define measurable monitoring parameters that help to detect early signs of wear or failure. Some of the common parameters to consider include vibration levels, temperature readings, pressure fluctuations, and oil quality analysis. You should also define the frequency of data collection and analysis. Choosing the right parameters helps collect accurate data essential for predictive analysis.
Proper installation and configuration of monitoring tools like IoT sensors, vibration meters, thermal cameras, flow meters, and pressure gauges ensure continuous or periodic monitoring of equipment performance. Integrating these tools with CMMS software helps seamless data flow and analysis. Similarly, correct calibration of these condition monitoring tools helps provide accurate and reliable data for effective analysis.
Data collection is the core of predictive maintenance. You need to gather historical data to identify past failure patterns, operational trends, and recurring issues. After that, compare this information with real-time data from IoT devices and ERP systems to create a comprehensive dataset. Now, apply statistical models or machine learning to understand normal operating ranges, detect anomalies and early warning signs, and identify failure trends.
Businesses should set condition thresholds and failure limits based on equipment performance data. It allows you to establish normal operating conditions, define acceptable performance ranges, and set critical points indicating imminent failure. When equipment data approaches or exceeds these thresholds, it triggers alerts, sends automated notifications, and activates automated maintenance actions to prevent potential failures.
For effective prediction of equipment failures, you should develop predictive models using analytical tools like data analytics platforms, machine learning algorithms, and statistical methods. These models gather historical and real-time equipment data, analyze it, and identify key variables influencing equipment health. Such analysis allows businesses to forecast potential failures and intervene on time, reducing unnecessary maintenance.
Once you develop predictive models, define specific maintenance tasks triggered by predictive insights. It helps to identify potential failure types and causes and classify tasks based on urgency and risk level. You will also be able to plan and specify corrective actions like lubricating moving parts, inspecting electrical connections, cleaning or replacing air filters, and replacing worn bearings. It also ensures proactive response when equipment conditions approach critical thresholds, reducing downtime and disruption.
The next step is to create and optimize the maintenance schedule based on predictive insights and defined maintenance tasks. Predictive maintenance plans identify potential failure points, prioritize maintenance based on criticality, and develop maintenance timelines aligned with failure predictions. It helps to efficiently assign technicians, tools, and spare parts and adjust schedules based on real-time data and performance feedback.
Integrated predictive maintenance with CMMS for automated work orders, ERP software for resource planning and reporting, and IoT devices for real-time monitoring. Effective integration allows for automated task creation, real-time monitoring and alerts, and seamless documentation of all maintenance activities for improved workflow efficiency and comprehensive visibility into maintenance operations.
A successful predictive maintenance depends upon a skilled maintenance team capable of interpreting data and responding to predictive insights. The workforce must be provided training programs focusing on understanding predictive tools like sensors, monitoring systems, and analytics software, analyzing condition monitoring data and identifying critical trends, reacting to predictive alerts, and hands-on training for equipment diagnostics and condition monitoring. They must get opportunities for continuous learning to adapt to new technologies and updates.
To maintain the effectiveness of the predictive maintenance program, businesses must monitor KPIs like equipment uptime, failure rates, maintenance costs, and mean time between failures (MTBF). They should evaluate the effectiveness of the maintenance schedule, identify gaps, and update maintenance frequency and tasks based on KPI trends. They should continuously refine predictive models and strategies for better accuracy, cost-effectiveness, and alignment with business goals.
How does a predictive maintenance schedule differ from other types of maintenance?
Aspects | Predictive | Preventive | Condition-based | Reactive | Approach |
Predicts failures using real-time data from IoT sensors and analytics | Scheduled at regular time intervals | Triggered by real-time equipment conditions | Fix after failure |
---|---|---|---|---|
Trigger | Analyze real-time and historical data | Time-based or usage-based schedules | Specific condition thresholds being exceeded | Equipment breakdown |
Downtime Risk | Minimal (proactive prevention with early detection) | Moderate (planned downtime) | Low to moderate (based on detection accuracy) | High (unexpected failures) |
Cost Efficiency | Highly cost-effective with optimized maintenance planning | Moderate (risk of unnecessary maintenance) | Cost-effective with targeted interventions | High long-term costs (costly emergency repairs) |
Technology Requirement | High (IoT sensors, AI-driven analytics, predictive tools) | Low (simple scheduling systems) | Moderate (requires condition monitoring sensors) | Minimal (basic repair tools) |
What challenges businesses face when implementing a predictive maintenance schedule?
While predictive maintenance schedules offer numerous benefits, such as reducing downtime, lowering maintenance costs, improving equipment reliability, and extending asset lifespan, businesses are also prone to challenges like high upfront investment, inaccurate data, a gap in technical knowledge, and integration issues. These challenges, ranging from financial barriers to technical complexities, must be addressed to seamlessly implement maintenance activities.
- High Initial Setup Costs
- Data Collection and Accuracy Issues
- Technical Skill Gap
- Integration Difficulties with Existing Systems
Implementing a predictive maintenance program requires high initial setup costs. It includes investments in condition-monitoring equipment, such as IoT sensors, vibration analyzers, and predictive analytics software. Apart from equipment, businesses also need to invest in system integration, staff training, and IT infrastructure upgrades to handle large volumes of real-time data. These costs can be a financial burden for SMBs with limited budgets.
One way of overcoming high investment costs is to adopt a phased implementation strategy, where emphasis can be given to critical equipment for the highest ROI. Another countermeasure is to retrofit existing equipment with modern IoT sensors. It reduces the need for the purchase of new equipment, reducing operational costs.
Predictive maintenance relies heavily on accurate, real-time data, but comes across challenges like a lack of reliable historical data, creating a significant hindrance to establishing precise predictive models. Inconsistent or poor-quality sensor data due to calibration errors, environmental interference, or sensor degradation over time can lead to false alarms or missed failure predictions. It affects the reliability of the maintenance schedule.
Data accuracy can be maintained by investing in robust data collection systems that bring high-quality, calibrated sensors to the business. Likewise, implementing regular data validation procedures also ensures accuracy. Also, using edge computing helps to process information closer to the source, improving data while reducing latency and transmission errors.
Another obstacle that many organizations face is the technical skill gap. Implementing a predictive maintenance schedule requires specialized knowledge in predictive analytics, condition monitoring, and advanced maintenance technologies, which many traditional companies lack. They also experience the shortfall of expert personnel. Such situations require them to hire data scientists and specialists, adding a massive amount to the operational costs. Training existing employees for the operation and management of advanced machinery becomes a time-consuming and resource-intensive task for companies.
To overcome this technical skill gap, businesses must invest in targeted training programs to upskill the current workforce on maintenance technologies and data analysis techniques. They should collaborate with external consultants who specialize in predictive maintenance and implement user-friendly predictive maintenance software for simplified data interpretation.
Businesses also face the hindrance of integrating predictive maintenance tools with existing systems, such as traditional CMMS or ERP platforms. The main problem occurs due to incompatibility between new predictive technologies and older equipment, leading to data silos, isolating critical maintenance data within specific departments or systems. Integrating predictive analytics into the existing system requires custom APIs or middleware solutions, which make the process more sophisticated. These challenges result in inefficient workflows, manual data entry errors, and incomplete insights, hampering the effectiveness of maintenance strategies.
Organizations should opt for predictive maintenance software that offers open architecture and strong API support to ease integration issues. It ensures compatibility with legacy systems. Another solution involves conducting a system audit to identify potential integration issues early. Businesses should work with integration specialists for tailored solutions for seamless data flow.
How to Use Predictive Maintenance Software to Schedule Maintenance Tasks?
Predictive maintenance software transforms maintenance management from reactive to proactive, data-driven. It automates data collection through IoT sensors and condition monitoring tools, gathering real-time data on vibration, temperature and pressure. This data is then analyzed through advanced algorithms and predictive models to identify trends, detect anomalies and forecast potential failures before they happen. As a result, businesses can create dynamic maintenance schedules based on the actual condition of the equipment and perform maintenance only when necessary to prevent costly breakdowns.
One of the key benefits of predictive maintenance software is its integration with Computerized Maintenance Management Systems (CMMS) which allows for automated tasks, real-time alerts, and streamlined workflows to enhance operational efficiency. By combining predictive with CMMS functionality, maintenance teams can manage work orders, track asset health, and allocate resources from a single platform. Also, real-time alerts allow rapid response to potential issues, minimize unplanned downtime, and reduce the risk of major equipment failures.
Tips for Effective Software Setup and Task Management
- Identify high-value or failure-prone assets.
- Set condition thresholds and define limits for vibration, temperature, and pressure.
- Automate work orders to enable auto-generation of tasks based on alerts.
- Integrate with CMMS to sync data for seamless data flow and task management.
- Customize alerts based on issue severity to prioritize urgent tasks.
- Update predictive models to regularly refine predictive algorithms with new data.
- Train staff to interpret predictive data and handle alerts.
- Review KPIs and track important metrics.